Computing minimal Markov bases
A Macaulay2 package to compute all minimal Markov bases
This project is joint with Ollie Clarke where we wrote the allMarkovBases package for Macaulay2 and the write-up which is now on the arXiv, which allows for the computation of all minimal Markov bases of a given integer matrix. The package builds on functionality of FourTiTwo by producing the fiber graph of the matrix. This graph allows us to compute the indispensable set of binomials as well as the universal Markov basis.
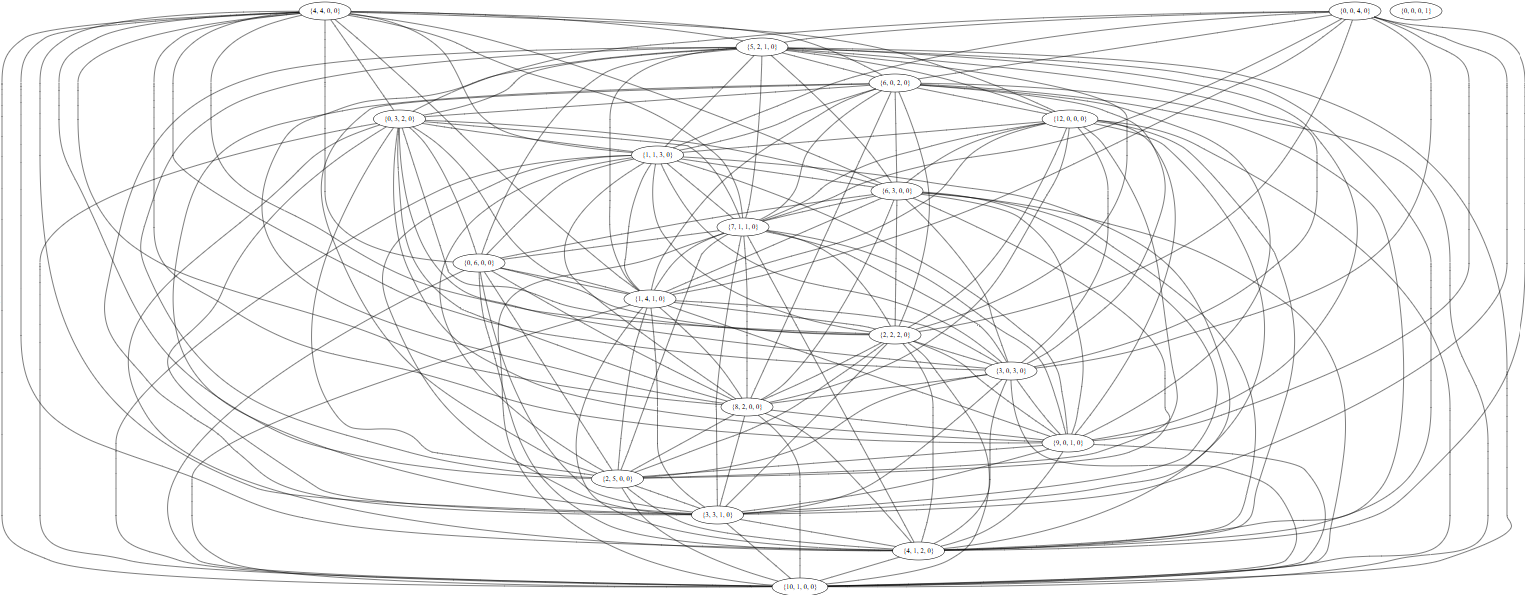
Introduction
Let \(k\) be a field. Given a configuration matrix \(A \in \mathbb{Z}^{d \times n}\) such that \(\ker(A) \cap \mathbb{N}^n = \{0\}\), its corresponding toric ideal \(I_A \subseteq k[x_1, \dots, x_n]\) is the ideal generated by the binomials \(x^u - x^v\) where \(u,v \in \mathbb{N}^n\) such that \(u - v \in \ker(A)\). Note that here we use multi-index notation, so \(x^u\) denotes the monomial \(x_1^{u_1}x_2^{u_2} \cdots x_n^{u_n}\).
A Markov basis \(M\) of \(A\) is a set of generators of \(I_A\) and is minimal if no proper subset of \(M\) generates \(I_A\). The seminal paper of Diaconis and Sturmfels (Diaconis & Sturmfels, 1998) provides a correspondence between the theory of Markov bases and Algebraic Statistics. The research landscape of Algebraic Statistics has seen a great deal of development in recent years, particularly in the study of Markov bases. For an introduction to the association of Markov bases to statistics, see (Kosta, 2020), or, for a more comprehensive overview, see (Almendra-Hernández et al., 2024).
The allMarkovBases Package
Macaulay2 already contains a package, FourTiTwo, which can compute a minimal Markov basis for a given configuration matrix however minimal Markov bases are almost never unique. The method markovBases from our package, allMarkovBases, builds on top of toricMarkov from FourTiTwo, incorporating Theorems 2.6 and 2.7 from (Charalambous et al., 2007) to compute all minimal Markov bases for a given configuration matrix. The package also includes the method randomMarkov where, given the NumberOfBases option, the method returns the given number of random minimal Markov bases. Both of these methods start by computing the fibers corresponding to the \(A\)-degrees of the minimal generators of the toric ideal \(I_A\) and this step of the computation can be done by itself using the fiberGraph method. The fibers are represented as graphs using the Graphs package in order to provide a visualisation of the fibers.
An Example with a monomial curve
We present an extended example to demonstrate the use of the package and some of the theory behind the code. Suppose our configuration matrix is the monomial curve \(A=(1, 2, 3)\). The first thing we might want to do is to compute the integer kernel of \(A\).
Macaulay2, version 1.24.11
with packages: ConwayPolynomials, Elimination, IntegralClosure, InverseSystems, Isomorphism, LLLBases, MinimalPrimes, OnlineLookup, PackageCitations, Polyhedra, PrimaryDecomposition, ReesAlgebra, Saturation, TangentCone, Truncations, Varieties
i1 : installPackage "allMarkovBases";
i2 : A = matrix "1,2,3";
1 3
o2 : Matrix ZZ <-- ZZ
i3 : ker A
o3 = image | 2 3 |
| -1 0 |
| 0 -1 |
3
o3 : ZZ-module, submodule of ZZ
Therefore, if we let \(x,y,z\) be variables, we know \(x^2 -y, x^3 - z \in I_A\). We might suspect that \(I_A := \langle x^u-x^v: u,v \in \mathbb{N}^3, u-v \in \ker_\mathbb{Z}(A)\rangle = \langle x^2 - y, x^3 - z \rangle\) and while the generators of the integer kernel lattice won’t in general be a Markov basis (see \(A=(3, 4, 5)\)), we can use the method toricMarkov from the FourTiTwo package to confirm that \(\{\{2,-1,0\},\{3,0,-1\}\}\) is a Markov basis for \(A\). Markov bases are outputted as matrices both in the FourTiTwo package and in our package.
i4 : toricMarkov A
o4 = | 2 -1 0 |
| 3 0 -1 |
2 3
o4 : Matrix ZZ <-- ZZ
We can notice that \(xy-z \in I_A\) and \(x^3-z \in \langle x^2-y,xy-z \rangle\) so \(I_A = \langle x^2-y,xy-z \rangle\) and thus \(\{\{2,-1,0\},\{1,1,-1\}\}\) is another Markov basis for \(A\). If we run the markovBases method from our package, we can confirm that these are in fact the only two minimal Markov bases for \(A\).
i5 : markovBases A
o5 = {| 2 -1 0 |, | 2 -1 0 |}
| 3 0 -1 | | 1 1 -1 |
o5 : List
One observation about the minimal Markov bases we have found is that the set of \(A\)-degrees is the same for both since \(\deg_A (x^2-y)=2\), \(\deg_A (x^3-z)=3\) and \(\deg_A (x^2-y)=2\), \(\deg_A (xy-z)=3\). Indeed, in general the \(A\)-degrees of a minimal Markov basis are independent of the choice of the minimal Markov basis, see (Miller & Sturmfels, 2004). Thus, we can look at the fibers corresponding to the \(A\)-degrees of any set of minimal generators of \(I_A\).
Thus, the fiberGraph method in our package starts by computing the \(A\)-degrees of the one minimal Markov basis computed by toricMarkov. Using a breadth-first-search algorithm, it constructs the fibers corresponding to \(A\)-degrees as graphs where two elements \(u,v \in \mathbb{N}^n\) of a fiber share an edge if they have non-trivial intersection ie. \(u_i>0\) and \(v_i>0\) for some \(i\).
i6 : fiberGraph A
o6 = {Graph{ {0, 1, 0} => {} }, Graph{ {0, 0, 1} => {} } }
{2, 0, 0} => {} {1, 1, 0} => { {3, 0, 0} }
{3, 0, 0} => { {1, 1, 0 } }
o6 : List
The fundamental theorem of Markov bases, see Theorem 3.1 in (Diaconis & Sturmfels, 1998), states that Markov bases are in 1-to-1 correspondence with sets of elements which connect any fiber corresponding to an \(A\)-degree. Thus, looking at the left graph from Figure 2, it is clear that \(x^2-y\) is an indispensable element ie. every Markov basis contains it. To connect the right graph from Figure 2, our Markov basis must contain either \(xy-z\) or \(x^3-z\) in addition to \(x^2-y\) and from what we calculated about \(I_A\), these form the only two minimal Markov bases of \(A\).
In general, we can use Theorem 2.6 from (Charalambous et al., 2007) to give us an algorithm to compute minimal Markov bases. For each fiber (which is represented as a graph with edges between elements which have nontrivial intersection) we treat the connected components as nodes of a new graph for which we choose a spanning tree. Then the union over all fibers of the binomials \(x^u-x^v\) where \(u\) and \(v\) are in distinct connected components connected by an edge of the spanning tree form a minimal Markov basis. Then Theorem 2.7 from (Charalambous et al., 2007) tells us that every minimal Markov basis can be found using the algorithm above.


Applying the algorithm to our example, the connected components of our fibers can be calculated by changing the option ReturnConnectedComponents
of fiberGraph to true
.
i7 : fiberGraph(A, ReturnConnectedComponents => true)
o7 = { { { {2, 0, 0} }, { {0, 1, 0} } }, { { {3, 0, 0}, {1, 1, 0} }, { {0, 0, 1} } } }
o7 : List
Thus, there are two connected components in each fiber as can easily be seen from Figure 2 and so there is only one possible spanning tree for both fibers (just the one edge between the two connected components). For the left graph, we also have no choice over which element of the connected components we choose as each connected component contains only one element, therefore, we end up with the indispensable element \(x^2-y\). For the right graph, the only choice we can make is which element \(u\) of the connected component with two elements \(\{\{1,1,0\},\{3,0,0\}\}\) we choose for the binomial \(x^u-x^v\) where \(v=\{0,0,1\}\). So, as expected, our algorithm produces two Markov bases, \(\{x^2-y,xy-z\}\) and \(\{x^2-y,x^3-z\}\).
The final non-obvious aspect of the algorithm is how to generate all the spanning trees on \(n\) nodes. For this, we use a result from Prüfer’s paper, (Prüfer, 1918), which states that there is a bijection between all spanning trees on \(n\) nodes and all sequences of elements \(\{1,...,n\}\) of length \(n-2\), known as Prüfer sequences. The paper also provides an algorithm to compute a spanning tree from a given Prüfer sequence.
Our package contains the pruferSequence method which takes in a Prüfer sequence and outputs the edge set of the corresponding spanning tree using the algorithm from Prüfer’s paper. For our example, for either fiber there are two connected components, so to calculate the spanning trees, we input the only Prüfer sequence for \(n=2\): the empty sequence.
i8 : pruferSequence {}
o8 = {set {0, 1}}
o8 : List
This gives us the trivial spanning tree on two nodes as expected.
Finally, our package contains the randomMarkov method which firstly calls the fiberGraph method in order to construct the connected components of each fibers. Then, for each fiber with \(n\) connected components, it generates \(n-2\) elements from \(\{1,...,n\}\) and uses the pruferSequence method to compute the corresponding spanning tree and then outputs the Markov basis computed from this spanning tree and randomly chosen elements from each connected component. This way, Markov bases can be randomly and uniformly selected.
i9 : B = matrix "1,1,2,4,21;2,4,4,15,41";
2 5
o9 : Matrix ZZ <-- ZZ
i10 : L = length markovBases B
o10 = 2904
i11 : elapsedTime (markovBases B)#(random L)
-- .239432s elapsed
o13 = | 22 3 0 -1 -1 |
| 21 -4 0 1 -1 |
| 2 0 -1 0 0 |
| 9 -1 17 0 -2 |
| 1 7 0 -2 0 |
5 5
o13 : Matrix ZZ <-- ZZ
i14 : elapsedTime randomMarkov B
-- .0280204s elapsed
o14 = | 16 3 3 -1 -1 |
| 19 -4 1 1 -1 |
| 2 0 -1 0 0 |
| 39 -1 2 0 -2 |
| 1 7 0 -2 0 |
5 5
o14 : Matrix ZZ <-- ZZ
Thus, if we take \(B= \begin{pmatrix} 1 & 1 & 2 & 4 & 21 \\ 2 & 4 & 4 & 15 & 41 \end{pmatrix}\), then it is almost 10 times quicker to use randomMarkov than to compute all minimal Markov bases and choose one at random.
In addition, if we wanted 3 minimal Markov bases of \(B\), we can use the NumberOfBases
option.
i15 : randomMarkov(B, NumberOfBases => 3)
o17 = {| 18 3 2 -1 -1 |, | 22 3 0 -1 -1 |, | 6 3 8 -1 -1 |}
| 13 -4 4 1 -1 | | 15 -4 3 1 -1 | | 1 -4 10 1 -1 |
| 2 0 -1 0 0 | | 2 0 -1 0 0 | | 2 0 -1 0 0 |
| 21 -1 11 0 -2 | | 13 -1 15 0 -2 | | 25 -1 9 0 -2 |
| 1 7 0 -2 0 | | 1 7 0 -2 0 | | 1 7 0 -2 0 |
o17 : List
And this is still much faster than computing all the Markov bases and picking some at random.
i18 : elapsedTime M = markovBases B; for i from 0 to 100 list M#(random L);
-- .248358s elapsed
i25 : elapsedTime randomMarkov(B, NumberOfBases => 100);
-- .0418593s elapsed
References
2024
- Markov bases: a 25 year update2024
2020
- Markov Bases of Toric Ideals: Connecting Commutative Algebra and StatisticsLondon Mathematical Society Newsletter, 2020
2007
- Minimal Systems of Binomial Generators and the Indispensable Complex of a Toric IdealProceedings of the American Mathematical Society, 2007
2004
- Combinatorial Commutative Algebra2004
1998
1918
- Neuer Beweis eines Satzes über PermutationenArchiv der Mathematischen Physik, 1918